Product
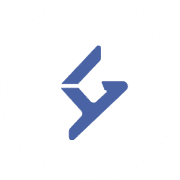
Genify
Jun 19, 2023
5 min read
What is a Transaction Categorizer?
A transaction categorizer is a service that analyzes transaction data, such as bank records or SMS messages and identifies the corresponding expense category that maps to this transaction. For example, for the transaction “Adidas, 100 USD, 2018–01–01”, the service looks for Adidas and identifies the business. and categorizes it as Sports and Shopping.
Transaction categorization is a service that is particularly useful for individuals and businesses as it provides insights into spending patterns and enables better financial management.
Comparing Transaction Categorizers
In this article, we will compare two prominent transaction categorizers, Genify and OneBrick, focusing on their geographical coverage, accuracy, sparsity, and latency. It is important to note that the experiments conducted in this article shed light on the challenges faced by local enrichment (categorization) engines when applied to global transaction data. These engines often rely on region-specific training data, which can lead to limitations in accurately categorizing transactions from different parts of the world. This article aims to explore this weakness by comparing two transaction categorizers, Genify and OneBrick, across multiple countries. The findings serve as valuable insight for users and developers seeking transaction categorization solutions for diverse global data.
Key Players in Transaction Categorization: Genify and OneBrick
1. Genify
Genify is a MENA-based company that offers personal finance management solutions (pfm) to banks and fintech. Their comprehensive solutions include spending analytics, event-based notifications, product recommendations, and alternative credit decisions. Genify aims to empower users with valuable financial insights and assist them in making informed financial decisions.
2. OneBrick
OneBrick specializes in developing financial APIs in Indonesia. Additionally, they make it simple for fintech businesses to digitally process financial data from end customers for each of their needs. This includes data from e-wallet, e-commerce, and super application transactions providing a seamless financial experience for users.
Below are details of the comparison we conducted between OneBrick and Genify.
Test Data
We compared the 2 services, Genify and OneBrick, regarding their geographical coverage, accuracy, sparsity, and latency.
To ensure a fair comparison between Genify and OneBrick, the experimental setup was carefully designed. The test dataset comprised randomly selected transactions from five different countries: the United States, the United Arab Emirates, India, Indonesia, and Egypt. The transactions were sourced from a wide range of merchants, randomly selected from each country’s top 10 retailers. By using this approach, the dataset aimed to capture a representative sample of transaction data from each region, mitigating potential biases. It is important to note that the experiments were conducted a month ago, providing a recent snapshot of the performance of both transaction categorizers.
Results
United States

Genify
Average Latency: 1852 ms
Unique Categories: 6
Unique Sub-Categories: 6
OneBrick
Average Latency: 1278 ms
Unique Categories: 1
Unique Sub-Categories: 1
United Arab Emirates

Genify
Average Latency: 1852 ms
Unique Categories: 6
Unique Sub-Categories: 6
OneBrick
Average Latency: 1278 ms
Unique Categories: 1
Unique Sub-Categories: 1
United Arab Emirates

Genify
Average Latency: 1986 ms
Unique Categories: 7
Unique Sub-Categories: 7
OneBrick
Average Latency: 1188 ms
Unique Categories: 1
Unique Sub-Categories: 1
India

Genify
Average Latency: 1938 ms
Unique Categories: 6
Unique Sub-Categories: 8
OneBrick
Average Latency: 1184 ms
Unique Categories: 1
Unique Sub-Categories: 1
Egypt

Genify
Average Latency: 2051 ms
Unique Categories: 8
Unique Sub-Categories: 9
OneBrick
Average Latency: 1261 ms
Unique Categories: 1
Unique Sub-Categories: 1
Indonesia

Genify
Average Latency: 2051 ms
Unique Categories: 8
Unique Sub-Categories: 9
OneBrick
Average Latency: 1261 ms
Unique Categories: 1
Unique Sub-Categories: 1
Indonesia

Indonesia’s results
Note: While testing OneBrick we discovered some test samples that generated results, so we added new test data.

Genify
Average Latency: 2051 ms
Unique Categories: 6
Unique Sub-Categories: 6
OneBrick
Average Latency: 1308 ms
Unique Categories: 3
Unique Sub-Categories: 4
Overall Category Sparsity
Genify
Unique Categories: 30
Unique Sub-Categories: 39
OneBrick
Unique Categories: 4 (food & dining, Shopping, Entertainment, transfer-out)
Unique Sub-Categories: 5 (Restaurants, hotel & vacation, Groceries, General, others account transfer-out)
Conclusion
Genify
Based on the results obtained from testing in five different regions, Genify’s API showcased its effectiveness by covering 30 unique categories and 39 unique sub-categories. It achieved an overall accuracy of 78% with an average latency of 1975 ms.
OneBrick
Our tests for OneBrick were only able to get results from Indonesia and OneBrick covered 4 categories and 5 sub-categories, demonstrating an overall latency of 1243 ms. It’s worth noting that OneBrick’s categorization was primarily based on keywords present in the transaction description and it was only able to categorize when the input included a word that maps to a category (e.g., Seafood Restaurant ➡️ Food & dining).
It is worth noting that OneBrick demonstrated promising performance in categorizing transactions, particularly in Indonesia. The OneBrick engine effectively mapped transaction keywords to relevant categories, providing accurate results within a competitive latency range. However, its relatively limited performance sheds light on the challenges faced by local enrichment (categorization) engines when applied to global transaction data as these engines often rely on region-specific training data, which can lead to limitations in accurately categorizing transactions from different parts of the world.
On the other hand, Genify demonstrated a robust performance across multiple tested regions. It provided broader coverage and a higher level of accuracy. For readers interested in testing Genify, the categorization service can be accessed via the website at https://www.genify.ai/txn-data-gui. Testing Genify firsthand will allow users to experience its functionalities and assess its suitability for their specific needs.
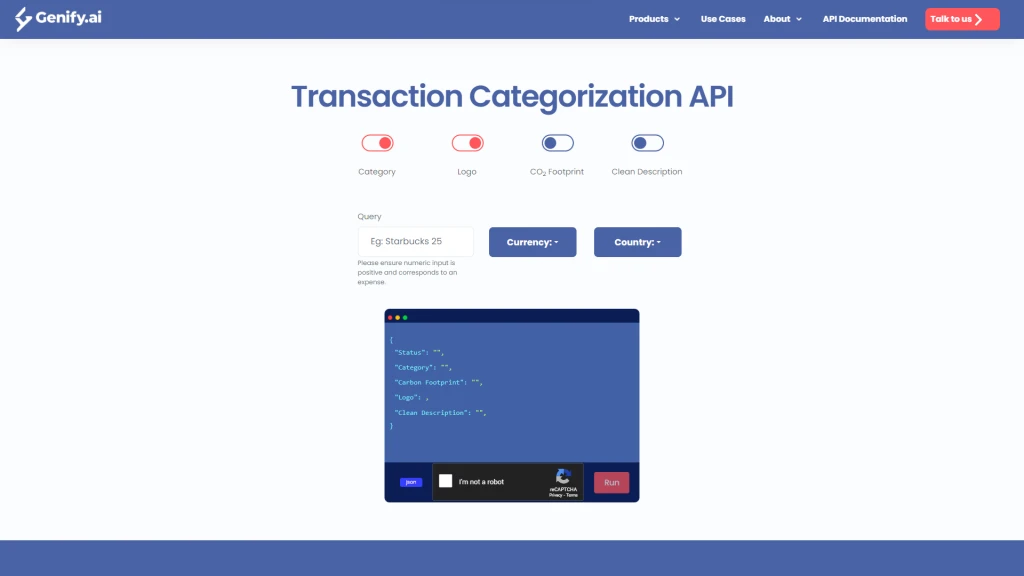
Ultimately, the choice between the two categorizers depends on the specific needs and priorities of users, taking into consideration factors such as geographical coverage, accuracy requirements, and the complexity of transaction data.
Sources
Test data: